This Is How We Use AI to Improve the Experience in an e-Commerce Website
On an e-commerce website, the comment box at the end of a purchasing process can be a valuable source of information and an exciting opportunity to personalize our products and services.
Until recently, obtaining valuable insights from the comment box was a complex process involving analyzing hundreds of thousands of natural language text entries. This task was even more difficult if we consider that the text in the comment boxes will often have colloquial uses or regional variants of the users’ language.
Next, we will talk about how, through a methodology that takes advantage of the analysis capacity of ChatGPT, we obtained insights with which we managed to improve the coherence between physical and digital shopping experiences while anticipating user needs and offering more personalized experiences
Step 0: Exploratory analysis in the field
Before reviewing the data we will work with, we should understand everything behind the purchase and delivery process in detail.
So, our process began with interviews that allowed us to understand the journey of critical stakeholders in the purchasing process, such as butchers, pick and packers, and area leaders.
This part of the process allowed us to delineate the role of the comment box and some of the nuisances associated with this order field.
In general terms, we can say that this step was fundamental to understanding where the nuisances were that prevented the experience of shopping through digital channels from being satisfactory or consistent with that offered by physical stores where, for instance, we can choose the ripeness of fruits and vegetables accurately.
Step 1: Preliminary analysis and filtering available information
As in many analysis processes, it is essential to guarantee the quality of the data with which we will work. In the case of comment boxes, there are many entries that we can discard from the beginning, either due to lack of coherence or because they do not contain information relevant to the order.
After this filter, a preliminary analysis can be done to identify the principal needs that users seek to satisfy when they write a message in the comment box.
For example, we can identify that requests frequently appear about the characteristics of a product, such as “I need the tomato ripe.” We can also find that messages about payment methods, such as “Please bring exchange for a 100,000 bill,” or the delivery process, such as “The doorbell is broken, please knock loudly,” are frequent.
This preliminary analysis can shed light on which products most frequently require clarification in the comment box. Later, we will see that this will allow us to establish personalization options we could offer users and how we should prioritize them to improve the shopping experience.
Step 2: Ontology construction
The previous step helps us define the main categories or themes of the requests we are working with and the main keywords people use to describe their preferences. From this information, we can build an ontology, which is a set of terms related to each other.
For example, we can identify that in their comments, customers write texts related to the desired “ripeness” of a product, such as “half-ripe or unripe tomato.” Thus, the ontology identifies a “mother” concept, such as the “ripeness,” and several child terms: ripe, unripe, half-ripe, half-unripe, etc.
The systematic construction of the ontology serves as input for the “prompt” given to ChatGPT so that it can follow precise instructions that help it tag the large volume of customer comments.
Of note is that for ontology to work efficiently, it must be able to consider the cultural and linguistic nuances in the messages we will analyze. Although we are using AI tools, we are also talking about a process in which human participation is essential. It will not only help establish the hypotheses and rules that will shape the ontology but must also identify keywords and other fundamental elements so that AI can make precise analyses.
Step 3: Creating a prompt in ChatGPT
With the previous step, we built the base and primary input to give ChatGPT the ability to categorize order remarks efficiently and in a structured way.
To build the prompt, we followed these steps:
- Role definition: We instructed ChatGPT to act as an “order remarks categorizer.” This is especially useful for giving the tool more context and increasing accuracy in its responses.
“You are a categorizer of order remarks.” - Use of constructed ontologies and instructions for categorization: The tool was told how to tag each comment with the previously completed ontology. We also point out that each may fit more than one category and that comments that do not check the predefined categories should be tagged “other.”Finally, we gave it specific instructions, so it did not mention any particular product in the answers, only the categories previously provided.
“Please categorize the following list of remarks according to the following tags and sub-tags. Note that each observation can be classified with multiple tags and sub-tags. If a comment does not fit any specified tags or sub-tags, tag it “Other.”
Tags and sub-tags:
For fruits and vegetables: ripeness, weight, size, quantity. For meat products: butterflying, slice, sliced, filleted, shredded, ground, whole, cut, skinless, weight, other.
General: indications, payment method, expiration date, purchase, and pick up.
Please only tag each observation with the appropriate tags and sub-tags (or “Other” if applicable) in the index of each comment WITHOUT mentioning any specific products.” - Exemplification of the categorization process: An example of what the final result should look like was provided to ensure that the categorization of observations was accurate. This step is crucial to clear up any ambiguities in the instructions.
For example: “20. Fruits and vegetables: ripeness, weight. Meat products: ground, sliced.” - Clarification of terms: ChatGPT needed to have context of terminologies used colloquially in different regions; through trial and error, we determined it was necessary to clarify the concept “ripeness” for fruits and vegetables as referring to physical characteristics such as color, texture, sweetness, etc.
“Keep in mind that the sub-tag “ripeness” on fruits and vegetables is related to terms that refer to physical characteristics of the product; for example, color, texture, hardness, sweetness, and NOT size.”
With this prompt, we managed to achieve the desired results.
Step 4: Pilot testing and estimating the margin of error
To calculate the error rate of ChatGPT analyses, we ran a pilot test. We randomly chose 10% of the total comments to be analyzed and tagged them manually.
Thanks to this process, we could check the differences between the tags of the comments made by a human versus the same comment tagged by ChatGPT, as shown in the following table:
This comparison allowed us to estimate that the AI error rate was small (less than 5%). It also showed us that the analysis method was viable for mass use.
We detected some adjustments we could implement in a second iteration to achieve even more accurate analysis.
Step 5: Obtaining insights and improving the experience
Using AI allowed us to increase analysis capacity while reducing the time we would have spent with other methods by nearly 50%.
It also gave us clear information about what to prioritize in the personalization process. The analysis lets us know which products appear most frequently in the comment box and which specifications users request the most.
For example, by analyzing thousands of messages in the comment box, we could discover that meat products have many more specific requests from users and that “skin” or “skinless” is vital within these requests or preferences. With this information, we can make specific changes to the shopping experience.
The analysis also helped us detect and resolve other common problems in digital orders, such as confusion when a user adding three product units receives 3 pounds instead.
Companies often know they must strengthen their personalization processes but are unclear where to start. This methodology shows how unstructured information from a tool like the comment box, combined with human talent and AI tools, can quickly and effectively solve this problem.
Please contact us if you want Pragma experts to help you personalize your digital assets.
Share this
You May Also Like
These Related Stories
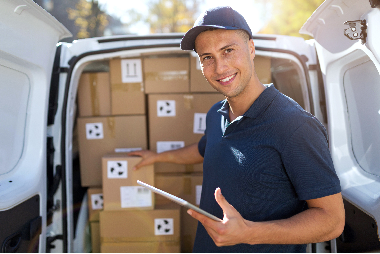
Last Mile Delivery: This is How AI Can Help To Overcome Its Challenges
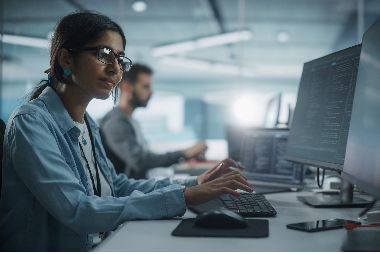
Three Reasons to Adopt DevSecOps in Banking App Development
%20-%20Cloud%20service%20(en)/ebook-competencia-aws-publicidad-merketing-card.png?width=431&height=279&name=ebook-competencia-aws-publicidad-merketing-card.png)
Pragma Achieves the new AWS Advertising and Marketing Technology Competency
Subscribe to
Pragma Blog
You will receive a monthly selection of our content on Digital Transformation.
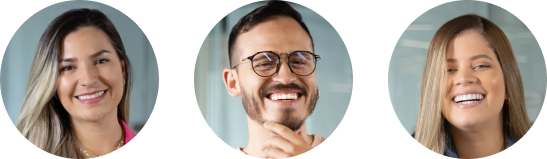