Data Architecture for Banking: A Guide to Success
Many companies in the financial sector have many data sources, which are vital for achieving their business objectives. Despite this, harnessing these resources’ true potential requires designing and implementing a data architecture that caters to each organization’s particular needs.
Over the years, Pragma has offered comprehensive services ranging from consulting and design to implementation and support for custom-built data solutions. Thanks to this experience, we have identified some challenges that companies face when seeking to obtain more value from their data:
- Lack of a clear data strategy: Many companies do not have a comprehensive data strategy to guide their data management and analytics efforts.
- Fragmented and disparate data: Data is often scattered across multiple systems and formats, making it difficult to integrate and manage centrally.
- Low data quality: Data quality is variable, with problems such as duplication, errors, and inconsistency affecting the reliability of data-driven analysis and decisions.
- Regulation and compliance: Companies must comply with regulations and compliance requirements related to data management and protection.
- Technology infrastructure inadequacy: Existing infrastructure cannot manage data efficiently, which may require significant investments in new tools and platforms.
One of our recent success stories clearly illustrates the above. Through strategic Data Warehousing, we enabled one of the most prominent neobanks in the region to accelerate reporting and establish rigorous data governance, allowing it to automate the processes of generating and transmitting legal reports for unions and guarantee funds.
Below, we will address some concepts and use cases to help you understand the best data architecture for your organization’s needs.
What is data architecture?
Data architectures are sets of principles, rules, and practices that provide a solid foundation for collecting, storing, processing, and analyzing data. They facilitate informed decision-making, improve operational efficiency, and promote innovation. Additionally, they help the organization become more competitive, provide better customer experiences, and meet regulatory requirements.
A good data architecture will not only influence our confidence regarding the available data; it is also essential to access the benefits of advanced data analysis or implement solutions based on Artificial Intelligence, a technology with many applications in banking and retail.
Data architecture also plays a fundamental role when it comes to improving the quality of information as it eliminates silos, avoids redundancy problems, and facilitates integration with new components and applications in the medium and short term.
What data architecture works best for my company?
Determining a company’s best data architecture depends on several factors, such as its business objectives, the volume and variety of data it handles, its available technological and human resources, and the regulations and compliance requirements it is subject to.
No single answer applies to all businesses, as each organization has unique needs and circumstances.
There are several data architectures, each with its characteristics and approaches. The most common types are Centralized, Distributed, Service-Oriented (SOA), Event-Oriented, and Microservices-Oriented. When talking about storing, integrating, analyzing, and managing data in business environments, you should know the difference between data lake, data warehouse, data lakehouse, data fabric, and data mesh.
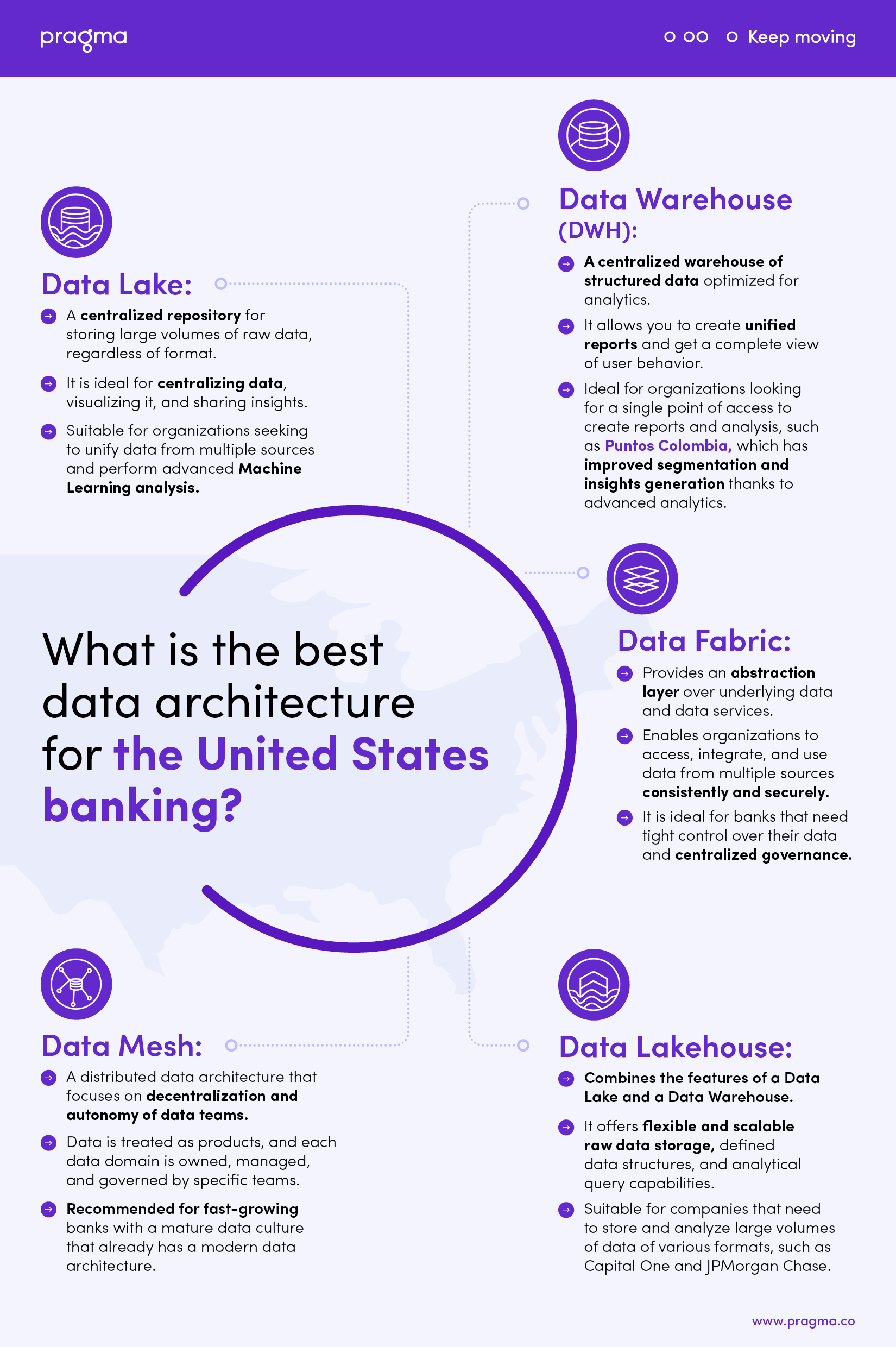
Data Lake
A data lake is a data repository that allows large volumes of data, whether structured, semi-structured, or unstructured, to be stored and consolidated in their original format.
In other articles, we have discussed tools that can simplify the creation and governance of data lakes, such as Amazon Lake Formation or S3. However, it is essential to remember that the cloud is also responsible for ensuring that these repositories are scalable and allow data exploration from multiple sources and formats.
Data lakes are perfect for organizations interested in the following:
- Centralize your data because they allow the creation of data ingestion processes that unify all sources of information.
- Take advantage of data visualization tools.
- Share analysis insights across business units.
- Implement advanced Machine Learning models.
Data Warehouse (DWH)
In contrast, a data warehouse is a centralized repository that stores structured data optimized for analysis. Through them, you can have the following:
- A single access point for creating reports
- A unified view of users that considers their behavior at all points of contact with the company
Another of our success stories is Puntos Colombia, a customer loyalty business formed by the bank and the country’s largest retail companies. At Puntos Colombia, we began by creating a data lake that later enabled the creation of a data warehouse. Today, they receive information from over 12,000 companies and over 6.3 million users. Thanks to advanced analytics, they have improved segmentation and generated insights.
Data Lakehouse
A Data Lakehouse is a data management platform that combines the characteristics of a data lake and a data warehouse.
This architecture provides flexible and scalable raw data storage while supporting defined data structures and analytical query capabilities.
By combining a data lake and a data warehouse in a single structure, companies can obtain:
- Low-cost storage for all types of data
- Standardized storage formats
- Administration features
- Real-time transfer and generation of statistics
- Support for scalable transactions and processing resources
Around the world, financial entities such as Capital One and JPMorgan Chase have used this type of architecture to store and analyze their data.
Data Fabric
A data fabric architecture would be the best option if you are looking for a data integration approach that provides a layer of abstraction over the underlying data and data services. It allows organizations to access, integrate, and use data from various sources consistently and securely.
A Data Fabric architecture may be more suitable for:
- Banks that need strict control over their data
- Financial institutions requiring centralized governance and large-scale data integration
Data Mesh
Finally, a data mesh is a distributed architecture focusing on data teams’ decentralization and autonomy. In a data mesh, data is treated as products, and each data domain is owned, managed, and governed by specific teams. This enables greater agility and scalability in data management as teams can iterate and evolve their data products independently.
This type of architecture is recommended for:
- Rapidly growing banks
- Banks with a mature data culture
- Banks that already have a modern data architecture, that is, they already have data lakes, DWH, and data integration platforms.
Remember that migrating to a data mesh can be complex and time-consuming, so it is essential to carefully evaluate your needs, capabilities, and best partner before deciding to migrate.
To learn more about Pragma’s experience implementing these types of architecture and the proprietary methodologies we have developed with each project to improve data architecture, governance, and analysis, we invite you to read more about our AWS data and analytics competency.
Are you seeking a partner to take your organization’s analytical maturity to the next level?
Share this
You May Also Like
These Related Stories
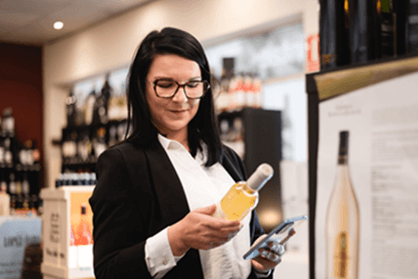
Data Lake on AWS for Data Management at Dislicores
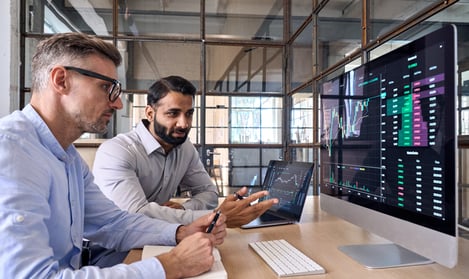
How to Improve Your Data Management? Data Warehouses vs. Data Lakes
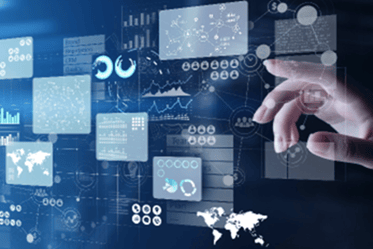
Data Science for Business
Subscribe to
Pragma Blog
You will receive a monthly selection of our content on Digital Transformation.
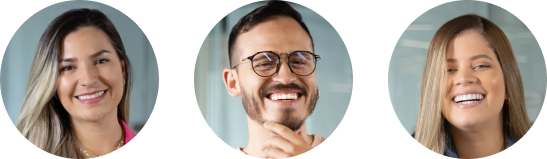