Predictive Analytics: The key to Hyper-Personalization in banking
As consumers demand experiences increasingly tailored to their needs and preferences, financial institutions must evolve to offer solutions beyond basic personalization. Hyper-personalization is an advanced approach that integrates real-time data, artificial intelligence, and predictive analytics to design unique products and experiences for each customer.
Next, we will explore the key aspects of hyper-personalization, how it differs from traditional personalization, its connection with predictive analytics, and some practical tips for implementing it in the banking sector.
What is Hyper-personalization, and Why Is It Essential for Banking?
Hyper-personalization refers to the accurate and real-time adaption of products, services, and communications to each user’s unique needs.
Unlike traditional personalization, which creates segments grounded in demographic data, hyper-personalization is based on a wide variety of data from physical and digital channels, from which it is possible to know each user’s preferences.
Hyper-personalization goes far beyond using the customer’s name in an email or text message. Rooted in users’ consent to process their data, hyper-personalization allows for establishing real bonds and an in-depth understanding of customer preferences to offer timely solutions that accurately fit their needs.
For example, using geolocation data, a bank could send a discount offer to a store when the customer is close to it. Additionally, through predictive analytics, financial institutions can improve their offering with products that meet customer expectations and are made available at the right time.
From a pre-approved loan in the bank’s app when the customer needs it to personalized insurance recommendations based on their consumption habits, hyper-personalization can significantly improve customer experience and conversion rates.
The Role of Predictive Analytics in Hyper-personalization
Hyper-personalization is only possible with advanced technological abilities that have emerged in recent years, one of which is predictive analytics.
Predictive analytics processes large volumes of historical data to identify patterns and predict events through data mining, advanced statistics, machine learning, and artificial intelligence, among other techniques. This does not imply having a “crystal ball,” but it does enable financial institutions to make decisions based on insights.
A bank, for example, can detect when a customer might be interested in a specific financial product, such as a mortgage loan, a credit card with particular benefits, or travel insurance. It can also identify the best time to offer these products, ensuring appropriate user interactions.
Predictive analytics allows us to customize offers and assess credit risk more comprehensively. Traditional models rely on limited financial data, such as credit history. Analytical models, meanwhile, can go further when considering a much broader range of information, such as spending patterns, payment behaviors, and other relevant signs.
Critical Tasks to Implement Predictive Analytics in Banking
For predictive analytics to be successfully implemented and hyper-personalization achieved, several vital tasks must be performed:
- Unification of information: Banks often have data dispersed across multiple business units, such as sales, marketing, and customer service. The first step is to unify this data into a single source of information, such as a Customer Data Platform (CDP) or Data Lake. This allows for a complete and consolidated view of the customer, essential for accurate predictive analysis. This centralized repository should include historical information, transactional data, cross-channel interactions, and stated or implied user preferences.
- Data transformation into valuable insights: Once the data is unified, it is crucial to transform it into actionable information. This involves applying machine learning models and predictive algorithms to identify recurring patterns and behaviors. This process will enable banks to understand their customers better and anticipate their needs. It is important to have teams of trained data analysts and appropriate technologies to ensure data is processed efficiently.
- Trigger implementation and automation: The next step is drawing up business rules that activate automatic actions based on predictive insights. Suppose analytics suggest a customer is at the ideal time to receive a credit offer. In that case, the system should be able to automatically generate that offer and present it in the most appropriate channel, either through an in-app notification, a text message, or an email. This level of automation streamlines processes and ensures that offers arrive at the right time.
At Pragma, we are experts in developing technological solutions that utilize data to strengthen the relationship between financial institutions and their users.
Are you looking for a partner to implement predictive-analytics and offer experiences that meet each customer’s needs?
Share this
You May Also Like
These Related Stories
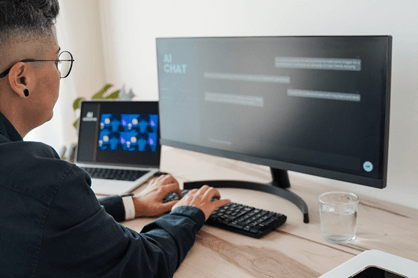
Intelligent Agents: Unlock Growth with Generative AI
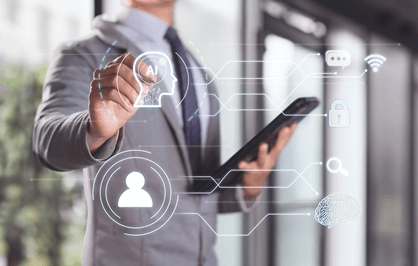
Generative AI: Transforming the Insurance Industry
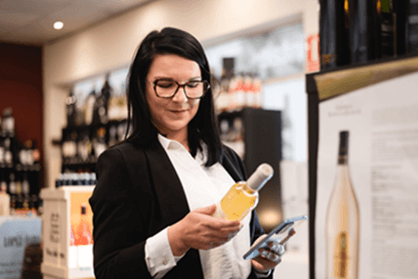
Data Lake on AWS for Data Management at Dislicores
Subscribe to
Pragma Blog
You will receive a monthly selection of our content on Digital Transformation.
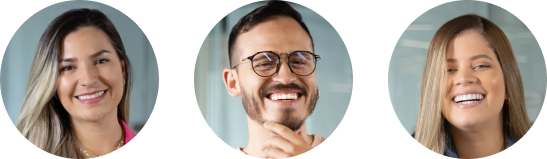