Five Myths Preventing Financial Institutions to Become Data-Driven
A myth is a belief that can limit our perception and hinder our progress. In the financial field, these myths can create perceptions that distort our vision of reality, lead us to error, and, more seriously, prevent us from building organizations where each decision is made objectively based on data analysis.
It is precisely for this reason that this article will explore five myths that we must dispel if we want to move forward efficiently on the path to having a data-driven organization where data and its accurate and rigorous analysis guide us to get to our business objectives faster.
Myth 1: “The number of models is what matters”
Many financial organizations believe that having many analytical models is the path to effective decision-making. However, this approach has significant limitations.
The power of our data strategy is not measured by the number of models or the size and specialization of the team of experts working on data analysis. For data to truly transform the financial sector, our models must produce a real and significant impact, which we can only achieve when we align our analytical strategy with the organizational vision.
More than quantity, what matters when we talk about data is the impact we can have on the lives of our end users and organizations.
Myth 2: “Data analytics is a race, and whoever comes first is inevitably the best”
The obsession with being at the forefront can lead financial organizations to seek prescriptive models and apply artificial intelligence without a clear understanding of the fundamental needs that will be met through these technologies.
Instead of running for the latest, we must realize the need and the solutions we generate through each model. This clarification shows that we will not always need complex predictive solutions. In many cases, a simple descriptive model can be enough to obtain valuable information and make correct decisions that help us achieve significant results by efficiently using our resources.
The key is understanding the science and purpose behind each model and applying them based on the business’s specific needs.
Myth 3: “Data governance can wait”
One of the most common mistakes when discussing a data strategy is postponing everything related to governance and focusing only on accumulating information.
The problem with this approach is that data governance with clear policies and guidelines, which are also rigorously followed, is essential to guarantee the quality and accuracy of our analyses. For this reason, we have seen that successful data strategies integrate data governance from the moment the system architecture is considered.
Ignoring the efforts that must be made to establish robust data governance can become a problem that grows over time and can negatively impact the effectiveness of an organization’s decisions.
Myth 4: “The single view of the customer is the responsibility of a single area”
Financial organizations often leave the responsibility of understanding the customer to marketing.
However, to be data-driven, constructing a 360 view of the customer must be cross-cutting and based on multiple sources of information.
Understanding habits, preferences, and needs requires sociodemographic data and knowledge that transcends areas. Therefore, knowing our customers and understanding their needs must be considered a comprehensive strategy involving the entire company, ensuring that each interaction meets specific requirements.
This principle is the foundation of personalized services. Data analysis allows us to reach customers at the right time and with the product and service offering that caters to their needs.
Myth 5: “Technology based on personal knowledge”
Believing that technology should be based on personal preferences or supplier relationships can limit an organization’s possibilities.
Very often, we believe that we should “marry” a single technology, but in reality, the best solutions, those that best fit the needs of the business and serve to achieve objectives optimally, take advantage of the strengths of multiple technologies and suppliers to build a tailored solution.
Technology selection should focus on finding the most efficient solution that fits the organization's needs, regardless of individual preferences.
Conclusion:
Data-driven decision-making allows us to understand our customers better, optimize processes, and gain competitive advantages.
Burying these myths is essential to building data-driven organizations in the financial industry. It is time to challenge long-held beliefs and lead the transformation toward a culture of analysis and decision-making based on solid and strategic data. Thus, we will be able to emerge in the financial industry, transforming the lives of our users and rising to the challenges of an increasingly competitive world.
Share this
You May Also Like
These Related Stories
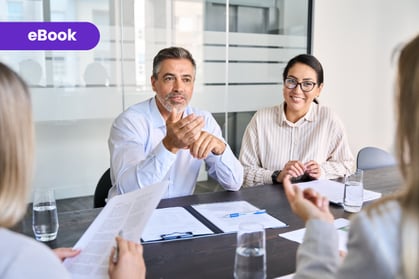
Unlock the Potential of Forecast Models in the Industry
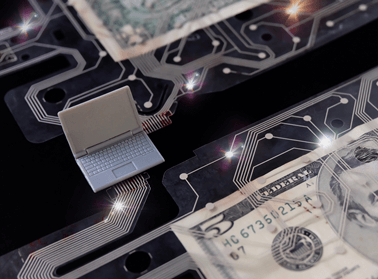
AI for compliance in banking: Role in fraud risk reduction
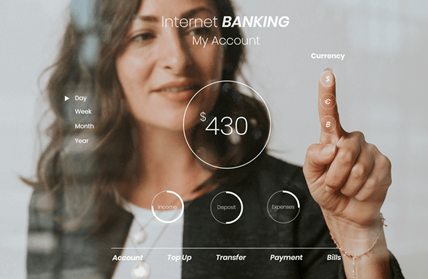
US Open Banking Outlook: Adoption, Benefits & Implications
Subscribe to
Pragma Blog
You will receive a monthly selection of our content on Digital Transformation.
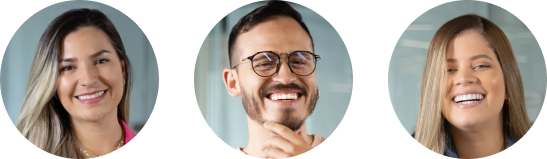